In the global economy and society, there is hardly an area in which artificial intelligence (AI) and machine learning (ML) have not yet found their way into – whether in mobility in autonomous driving, in the production of goods in the context of Industry 4.0, or in face recognition on Facebook. Companies optimize their business processes through AI and the modern working world is also increasingly characterized by intelligent systems that can help us and learn from people..
AI and ML are important drivers of digital transformation. In Germany alone, thanks to networked and automated production, productivity increases of around 78 billion euros can be achieved by 2025 in six relevant sectors of the economy, including mechanical engineering, automotive and chemicals, according to the President of the Fraunhofer-Gesellschaft, Prof. Reimund Neugebauer. Part two of the TUP series KI therefore deals with the term machine learning.
Fundamentals of Machine Learning
Machine Learning uses powerful algorithms to learn from huge amounts of data. A computer program analyzes examples and uses the self-learning algorithms to try to recognize certain patterns and methods in the mass of data. The selected software is supplied with ever new data sets and subsequently receives feedback from the programmer, which the algorithm uses to refine the resulting model. The larger the data volume the algorithms can access, the more knowledge they acquire. Basically, ML distinguishes between two approaches for the preparation of the data sets – supervised and unsupervised learning. Ultimately, the goal of machine learning is to intelligently link data, recognize correlations, draw conclusions and make predictions for new and unknown facts.
Machine learning is merely an umbrella term that describes the task of improving machines in solving a task by learning from data. This can also “only” refer to algorithms. The core is that the program or machine automatically adapts to new conditions and updates itself based on the available data.
Machine learning in intralogistics
Numerous companies are looking into the question of how intralogistics can be made even more efficient in the future. More effective processes require reliable master data on products and processes in order to exploit the potential of machine learning as much as possible. In the area of optimization of transport and order picking alone, the data volumes generated in intralogistics offer enormous potential for machine learning applications such as pattern and image recognition.
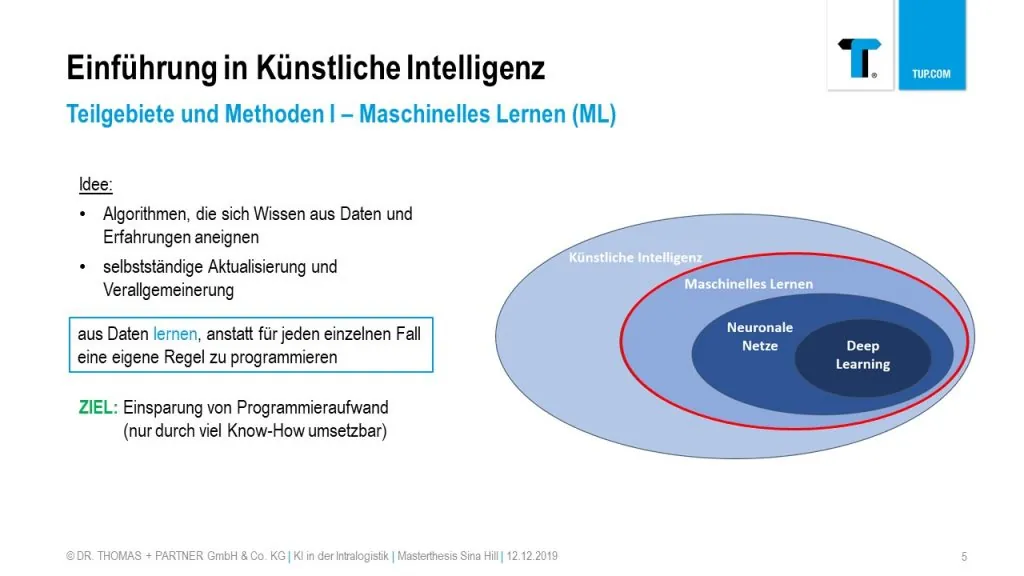
By using machine learning, material transports including loading and unloading times can be predicted much more precisely. Instead of setting lump-sum times, for example for transporting pallets, Machine Learning enables precise differentiation according to the products to be transported, with regard to scheduling and weekdays, while also taking into account the respective personnel situation. Machine Learning can thus help to organize processes much more efficiently and avoid possible bottlenecks. The situation is similar in the event of sudden disruptions in operating processes, for example, late deliveries. A self-learning system recognizes the necessities faster and can automatically de-escalate processes with suitable countermeasures.
Predictive maintenance offers a competitive advantage in highly optimized intralogistics
Advantages can also be realized through so-called ‘predictive maintenance’. By means of analytical ML applications, the logistician can determine maintenance and servicing dates for intralogistics technology and vehicle fleets and thus create more precise resource planning. As a result, machine downtimes can be reduced and costs for unexpected breakdowns can be cut. Predictive maintenance is not only important in the manufacturing industry, but is also becoming increasingly important for the main modes of transport (road, rail, air).
Furthermore, machine learning enables improved image recognition – a tool that enables mail order companies to maintain effective and error-free warehousing, especially in times of booming e-commerce. Scanning and recording processes form the basis of image recognition and monitor the fill level of the shelves or control the quality of the stored products. At the same time, they help locate materials or identify the location of machines and people, not only to work more efficiently, but also to prevent accidents within warehouses, for example.
Conclusion
Overall, self-learning algorithms appear to be the perfect solution for further increasing the efficiency of intralogistics and successively automating its processes. This creates the basis for a consistently digital and traceable supply chain – a key criterion for companies to increase delivery quality and operate more efficiently, according to the German government in its interim report „Ein Jahr KI-Strategie“ vfrom November 2019.